How Will AI Learn? Like We Do
I produced a special issue of Multiplex Magazine (Read: Twenty Questions In New AI) to address self-learning with Voice First AI systems. This issue showed just one way to solve what I call the log2(n) – n paradox (or the Evan’s paradox) that presents a postulation that computers could not possibly learn every question humans could ask. I presented the simple 20Q model to deal with the Fuzziness of humans, and present that it is not the “insolvable” problem that even learned experts suggest.
This week researchers at DeepMind the London-based artificial intelligence company owned by Alphabet Inc/Google solved the long term memory and neuron connections needed to maintain continuity systems called Elastic Weight Consolidation. Classic neural networks are based on the structure of synapses in the human brain. AI researchers have machine learning techniques for building a knowledge system. However neural networks suffered “catastrophic forgetting” at some point when the neuron density reached a critical level. Thus the state of most neural network systems were a perpetual present where every time the network is given new data, it would overwrite previous data.
~—~
~—~
DeepMind researchers drew on synaptic consolidation used in human and animal brains to create a way for neural networks to remember prior connections and neuronal states. Claudia Clopath, a neuroscientist at London’s Imperial College, a co-author on the paper helped the computer scientists better understand human models and the collaboration produced the paper: Overcoming catastrophic forgetting in neural networks [1], published in the academic journal Proceedings of the National Academy of Sciences.
Abstract:
The ability to learn tasks in a sequential fashion is crucial to the development of artificial intelligence. Until now neural networks have not been capable of this and it has been widely thought that catastrophic forgetting is an inevitable feature of connectionist models. We show that it is possible to overcome this limitation and train networks that can maintain expertise on tasks that they have not experienced for a long time. Our approach remembers old tasks by selectively slowing down learning on the weights important for those tasks. We demonstrate our approach is scal- able and effective by solving a set of classification tasks based on a hand-written digit dataset and by learning several Atari 2600 games sequentially.
Put simply, human brains create memories by connections between neurons. As the brain learns neurons wire together and fire together to reenforce the memory. Less important memories have less neuronal connections. The DeepMind researchers drew on this synaptic consolidation model to allow neural networks to remember.
Researchers tested the algorithm on ten classic Atari games, which the neural network had to learn to play from observations only. DeepMind had previously created an AI agent able to play these games equal to human players. however that earlier AI could only learn one game at a time. If it was later shown one of the first games it learned, it had to start all over again. Elastic Weight Consolidation enabled software was able to learn all ten games and, on average, come close to human-level performance on all of them. It did not, however, perform as well as a neural network trained specifically for just one game.
Elastic Weight Consolidation sets the importance of each neuron in a neural network to a task newly learned and assigns the neuron a mathematical weight proportional to importance. This weighting will slow down the rate at which the value of that particular node in the neural network can be altered. In this way, the network is able to retain the neuron knowledge while learning a new task.
In the special issue of Multiplex Magazine I explored how ultimately there are no limits to the questions one could ask a Voice First device and there are no limits to the answers using active learning and doing what humans do when they do not understand a question, ask for more detail. Asking for details with inductive and deductive self-learning using these new neuron building systems will rapidly move Voice First systems and their capabilities orders of magnitude farther ahead. It is ironic that just at the moment when some have suggested that universally useful Voice First devices will be quite limited and it is decades away for something satisfactory, a model for rapidly building neurons was just surfaced in this paper. With the 20Q model and Elastic Weight Consolidation, we will begin to see this in Voice Apps and Skills in months not decades
_____
[1] http://www.pnas.org/content/early/2017/03/13/1611835114.full.pdf
~—~
~—~
Subscribe ($99) or donate by Bitcoin.
Copy address: bc1q9dsdl4auaj80sduaex3vha880cxjzgavwut5l2
Send your receipt to Love@ReadMultiplex.com to confirm subscription.
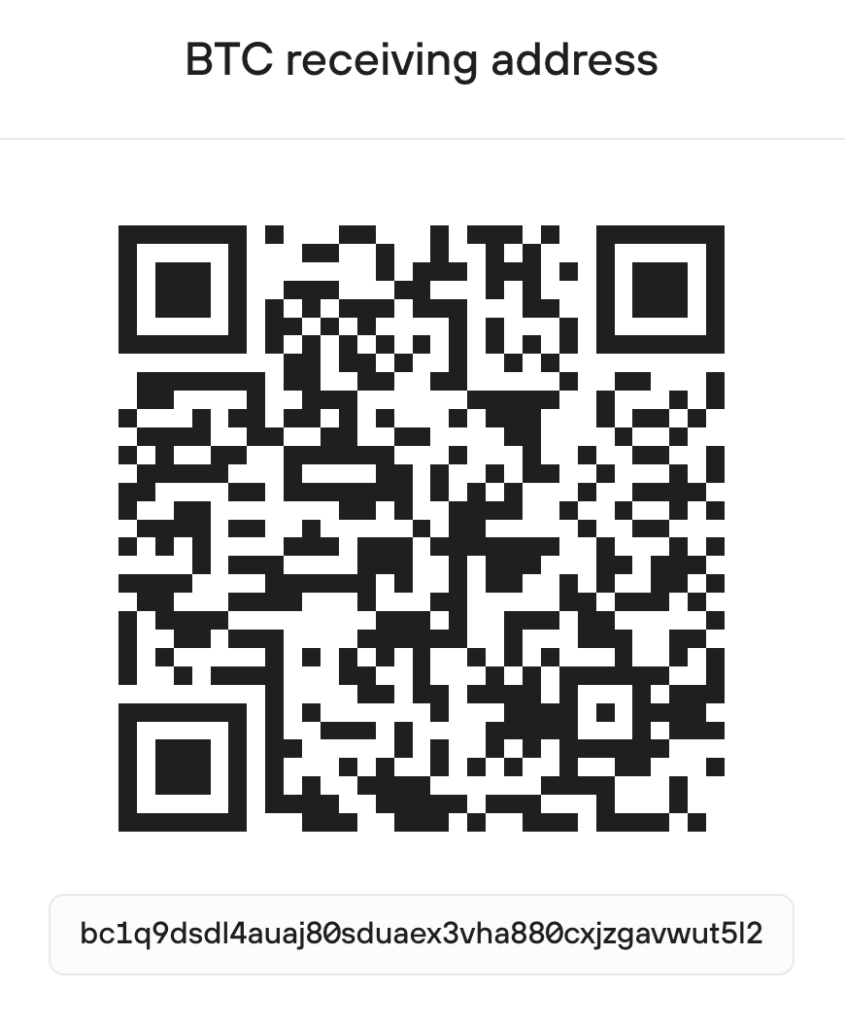
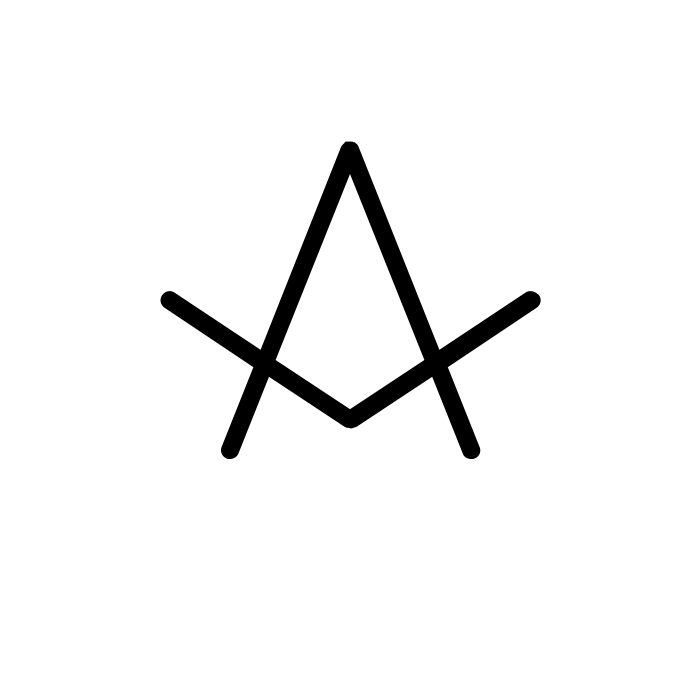
IMPORTANT: Any reproduction, copying, or redistribution, in whole or in part, is prohibited without written permission from the publisher. Information contained herein is obtained from sources believed to be reliable, but its accuracy cannot be guaranteed. We are not financial advisors, nor do we give personalized financial advice. The opinions expressed herein are those of the publisher and are subject to change without notice. It may become outdated, and there is no obligation to update any such information. Recommendations should be made only after consulting with your advisor and only after reviewing the prospectus or financial statements of any company in question. You shouldn’t make any decision based solely on what you read here. Postings here are intended for informational purposes only. The information provided here is not intended to be a substitute for professional medical advice, diagnosis, or treatment. Always seek the advice of your physician or other qualified healthcare provider with any questions you may have regarding a medical condition. Information here does not endorse any specific tests, products, procedures, opinions, or other information that may be mentioned on this site. Reliance on any information provided, employees, others appearing on this site at the invitation of this site, or other visitors to this site is solely at your own risk.
Copyright Notice:
All content on this website, including text, images, graphics, and other media, is the property of Read Multiplex or its respective owners and is protected by international copyright laws. We make every effort to ensure that all content used on this website is either original or used with proper permission and attribution when available.
However, if you believe that any content on this website infringes upon your copyright, please contact us immediately using our 'Reach Out' link in the menu. We will promptly remove any infringing material upon verification of your claim. Please note that we are not responsible for any copyright infringement that may occur as a result of user-generated content or third-party links on this website. Thank you for respecting our intellectual property rights.